LLMs And The AGI Question - A Technical Analysis Of Capabilities, Limitations, And Future Trajectories
As Large Language Models continue to advance, the pursuit of Artificial General Intelligence raises critical questions about technical feasibility and fundamental requirements. This analysis delves into the current state of LLMs, their limitations, and the potential pathways toward achieving AGI, highlighting the complex interplay between scaling, architecture, and novel approaches in AI development.
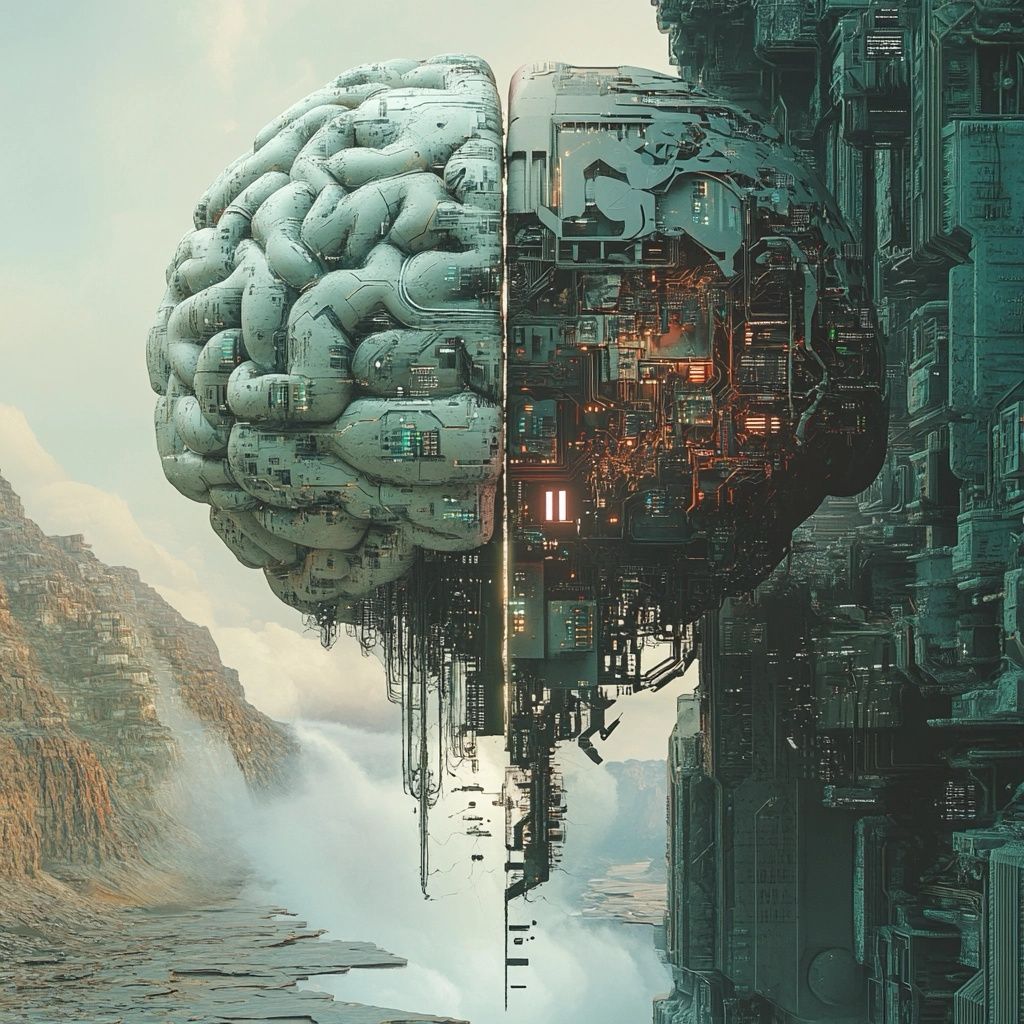
Mar 27, 2025
Large Language Models have transformed our technological landscape, generating human-like text and solving complex problems across domains. As these systems grow more sophisticated, a fundamental question emerges: Will LLMs eventually evolve into Artificial General Intelligence (AGI)?
This analysis examines the technical realities of current LLMs, the fundamental requirements of AGI, and whether we can bridge this considerable gap.
Understanding The Current LLM Architecture
Today's Large Language Models operate on predictive mechanisms, using transformer architectures to calculate probabilities of token sequences. They're trained on vast text corpora and optimize for next-token prediction. This fundamental design creates both remarkable capabilities and inherent limitations.
Modern LLMs like GPT-4, Claude, and others demonstrate impressive reasoning, planning, and knowledge application. However, they remain fundamentally statistical prediction systems without true understanding or agency. Their performance emerges from pattern recognition across massive datasets rather than conceptual understanding.
LLMs excel at:
- Recognizing and generating patterns in text
- Applying learned statistical relationships to new contexts
- Simulating reasoning processes through chain-of-thought mechanisms
- Solving problems within their training distribution
But they fundamentally lack:
- Intrinsic motivation and goal-directed behavior
- Grounded understanding of physical reality
- True causal reasoning capabilities
- The ability to independently verify information
The AGI Requirements Gap
Artificial General Intelligence requires capabilities beyond pattern recognition. True AGI would need:
1. Causal Understanding: LLMs can simulate causal reasoning but don't fundamentally understand cause and effect. They generate plausible-sounding explanations based on statistical patterns rather than actual causal models of the world. AGI would require genuine causal reasoning - understanding not just correlations but why and how things happen.
2. Embodied Intelligence: Humans develop intelligence through physical interaction with our environment, creating grounded understanding. LLMs lack this embodiment, operating solely in the symbolic domain of language. They have no direct experience with physical reality, resulting in what philosophers call the "symbol grounding problem" - they manipulate symbols without connecting them to real-world referents.
3. Self-Directed Learning and Agency: Current LLMs remain passive systems that respond to prompts. They don't independently set goals, seek information, or pursue understanding. AGI would require intrinsic motivation and the ability to direct its own learning processes autonomously.
4. Integrated Multimodal Understanding: While multimodal models can process different information types (text, images, audio), they still lack a unified understanding across modalities. True AGI requires seamless integration across perception systems and the ability to develop consistent mental models across different inputs.
5. Meta-Learning Capabilities: AGI systems would need to learn how to learn - adapting learning strategies based on context and developing new frameworks for understanding novel domains.
Technical Paths Forward: Evolution Or Revolution?
The question becomes: can we evolve LLMs toward AGI, or do we need fundamentally new approaches?
The Scaling Hypothesis
Proponents of the scaling hypothesis argue that continued scaling of parameters, training data, and computational resources will eventually lead to emergent AGI capabilities. This view suggests that intelligence emerges at scale without requiring new architectural breakthroughs.
Evidence supporting this includes:
- Emergent abilities appearing in larger models that weren't present in smaller ones
- Increasing performance on reasoning tasks as models scale
- Improvements in self-correction and planning capabilities
However, skeptics highlight that:
- Many capabilities show diminishing returns with scale
- Fundamental limitations in training paradigms (prediction-only optimization)
- Conceptual gaps that may not be addressed by scale alone
The Hybrid Systems Approach
A more moderate view suggests combining LLMs with complementary systems to address their limitations:
- Integrating symbolic reasoning modules for logical operations
- Adding world models and simulation environments
- Implementing planning and verification systems
- Developing memory architectures that support long-term reasoning
- Creating embodied agents that interact with environments
This approach acknowledges LLMs as powerful components but not sufficient on their own for AGI.
The Revolutionary View
Some researchers maintain that AGI requires fundamentally different architectures than current LLMs. This perspective argues that prediction-based learning, regardless of scale, cannot address core requirements of general intelligence.
Alternative approaches include:
- Neuromorphic computing systems that more closely mimic biological brains
- Active inference frameworks based on free energy principles
- Causally-aware architectures that explicitly model intervention
- Self-organizing systems with intrinsic motivation
Technical Bottlenecks And Research Frontiers
Several technical challenges currently limit progress toward AGI:
1. The Alignment Problem: As models grow more capable, ensuring they remain aligned with human values becomes increasingly difficult. This challenge intensifies with systems approaching AGI capabilities.
2. Explainability and Interpretability: Current LLMs operate as "black boxes" whose internal operations remain opaque. True AGI would require transparent reasoning that humans can understand and verify.
3. Computational Efficiency: The computational requirements of modern LLMs grow exponentially with scale. AGI would require either orders-of-magnitude more efficient architectures or computational breakthroughs.
4. Knowledge Integration and Verification: LLMs struggle to maintain consistent factual knowledge and lack mechanisms to verify information. AGI requires reliable knowledge maintenance and the ability to distinguish facts from fabrications.
5. Transferability Across Domains: While LLMs show impressive in-context learning, they still struggle with transferring knowledge across distant domains - a core requirement for general intelligence.
Timeframes And Predictions
Experts remain divided on AGI timelines, with perspectives ranging from:
- The Optimist View: AGI could emerge from advanced LLMs within 5-10 years through continued scaling, architectural improvements, and integration with complementary systems.
- The Moderate View: True AGI remains 15-30 years away, requiring significant breakthroughs beyond current LLM paradigms.
- The Skeptical View: LLMs represent a different path altogether from AGI, and current approaches will not lead to general intelligence regardless of scale.
Conclusion - Evolution With Revolutionary Components
The most plausible path forward appears to be evolutionary development of LLMs complemented by revolutionary components addressing core limitations. LLMs provide a powerful foundation for language understanding and generation, but true AGI will likely require:
- Grounded models with physical world understanding
- Causal reasoning systems beyond statistical correlation
- Active learning mechanisms with intrinsic motivation
- Integrated memory and reflective capabilities
- Fundamentally new approaches to knowledge verification
Rather than asking whether LLMs will evolve directly into AGI, perhaps the more productive question is: how will LLMs contribute to the broader ecosystem of systems that might collectively achieve general intelligence?
What seems clear is that the impressive capabilities of modern LLMs represent a significant step forward in AI development, but the journey to true AGI remains long and will likely require multiple conceptual breakthroughs beyond simply scaling our current approaches.